The release of GPT 3.5 and the improvement of large language models (LLMs) has captured the imagination of many around the world, as people grapple with how artificial intelligence can impact our lives and work.
AI has the potential to modernize multiple areas in oncology drug development and clinical trial management. By leveraging machine learning and big data analytics, AI can facilitate faster, more precise processes that offer the potential for better patient outcomes. This post explores the potential benefits from using AI from drug discovery to NDA.
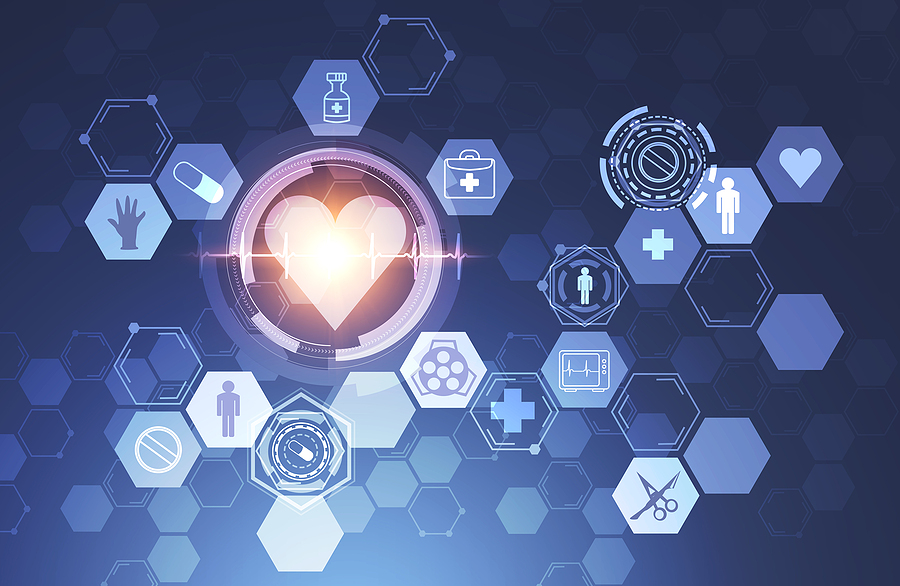
AI in Oncology Drug Discovery
In the initial stages of drug development, AI has proven to reduce cost and speed multiple processes:
- Acceleration of drug identification: AI algorithms rapidly sift through vast libraries of chemical compounds, predicting their efficacy against specific cancer targets based on historical and biological data. What used to take several years and billions of dollars in lab work, AI can accomplish in a fraction of the time and cost.
- Novel compound design: AI doesn’t stop at analyzing existing compounds; it can also generate suggestions for new chemical structures, expanding the pool of potential cancer drugs exponentially. Machine learning models can develop hypotheses based on existing data and ‘learn’ from past results, driving innovation in drug design.
AI in Preclinical Testing
AI’s role in preclinical testing allows for a more comprehensive understanding of a drug’s impact on biological systems:
- Predictive modeling: Machine learning models, trained on comprehensive genomics and proteomics datasets, can predict how a prospective drug might interact with different biological pathways. This capability helps minimize unforeseen side effects and the likelihood of drug resistance, leading to more effective treatments.
- High-throughput screening: AI algorithms can rapidly process, analyze, and derive insights from vast amounts of experimental data, allowing researchers to pinpoint the most promising drug candidates from among thousands of compounds, significantly speeding up preclinical testing.
AI in Clinical Trial Management
AI is transforming clinical trial management, improving efficiency, and precision:
- Enhanced patient recruitment: AI can analyze extensive patient databases, quickly identifying potential trial participants based on detailed medical histories. This ability not only accelerates patient recruitment but also improves trial diversity.
- Real-time monitoring and data analysis: AI can streamline the monitoring process, analyzing real-time data to quickly identify adverse effects, compliance issues, and treatment efficacy. This immediate feedback loop allows for more dynamic and responsive trial management.
- Trial design and risk management: AI can predict trial outcomes and potential challenges, informing more effective trial design and risk management strategies. By analyzing patterns in vast amounts of clinical trial data, AI can guide decision-making and increase the likelihood of trial success.
What’s Next?
AI is likely to have a significant impact in oncology drug development and clinical trial management. It facilitates the rapid identification and testing of potential new treatments, streamlines patient recruitment and trial management, and informs more effective trial design and risk management strategies.
As we continue to refine AI technologies and integrate them further into oncology research, the future of cancer treatment looks increasingly promising.
However, while AI offers significant potential, it’s important to continue rigorous evaluation of these tools. Ensuring that AI systems are transparent, reliable, and capable of functioning as intended is paramount. In the end, the goal is to augment human expertise with AI capabilities, enabling us to combat cancer more effectively than ever before.